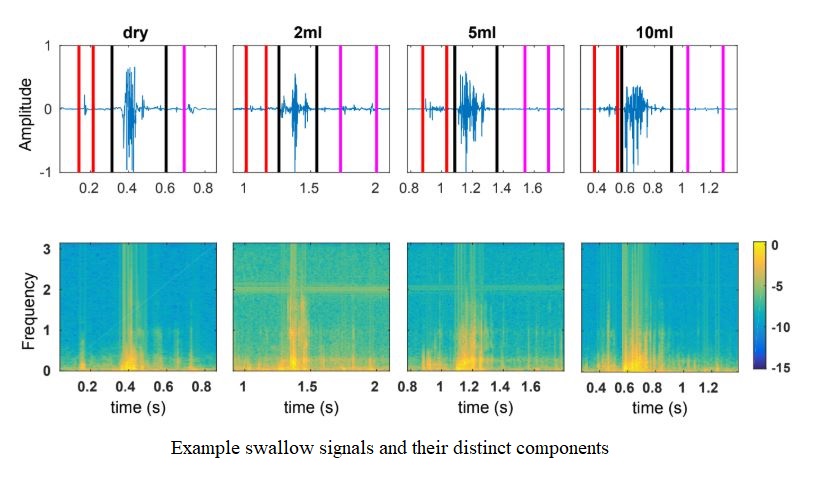
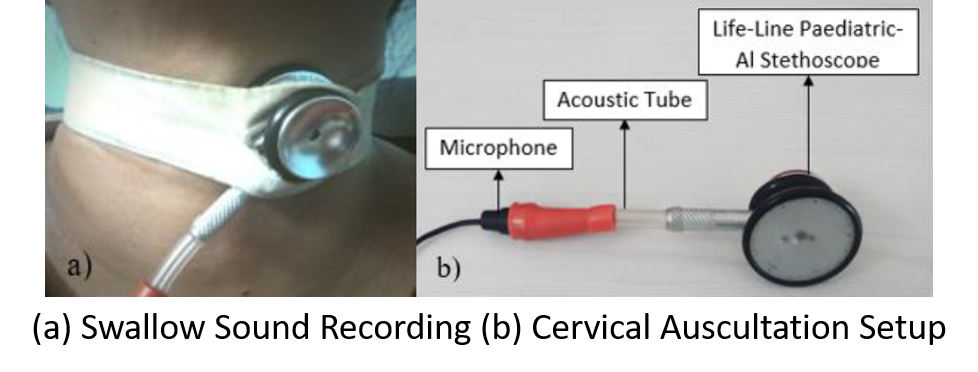
Patients with Head and Neck Cancer can experience dysphagia or more commonly known as swallowing disorders. Dysphagia can potentially lead to increased-risk medical conditions like pulmonary aspiration (both silent and overt), choking, fatigue and malnutrition. Such cases when unidentified can be fatal. The diagnosis of such disorders involves screening tests, and clinical assessments like fibreoptic endoscopy and videofluoroscopy. These techniques are invasive to the subject and can sometimes be harmful due to exposure to X-rays. Hence, in this project, the assessment of the physiology of swallowing is done using cervical auscultation (CA), where the swallowing action is captured by the sounds that are produced when the food bolus is swallowed. In CA, the sounds are picked up using an external stethoscope or microphone. The aim is to develop automatic methods to study the characteristics of swallow in both healthy and dysphagic subjects and also to detect the severity of dysphagia in Head and Neck Cancer patients, by leveraging signal processing and machine learning methods.
Initial work in this project involved feature learning for a volume dependent analysis and classification of water swallowed. Results indicated that, across different volumes, the acoustic features selected using automatic feature selection methods were more robust to volume changes than the baseline features that pertain to basic temporal and spectral parameters.
Project Members: Siddharth S, Achuth Rao M V
Collaborators: Prasanna Suresh Hegde, Health Care Global Enterprises Ltd.